Track: Data Science & Machine Learning Methods
Location:
- Whittle, 3rd flr.
Day of week:
- Wednesday
Data Science & Machine Learning Methods: How to start using machine learning and data science in your environment today. Latest and greatest best practices.
Modern businesses live or die by how savvy they are in putting their data to work, building using new data processing technologies but, more centrally, applying data science methods via those technologies to shape that raw material reliably into predictions, alerts, and business insights which gain the business an edge.
But effective data science programmes are complicated: creating powerful, sophisticated, and high impact solutions needs a mixture of software engineering, business understanding, and statistical skills, and is much more than just a one-time task.
In this track we will talk about data science and machine learning as they are implemented in real, modern businesses. We’ll look at the case studies, best practices, and pitfalls you need to know about as a developer, and we will see real stories of successful implementation of methods from the most commonly used (e.g. anomaly detection) to the most exotic (e.g. neural networks).
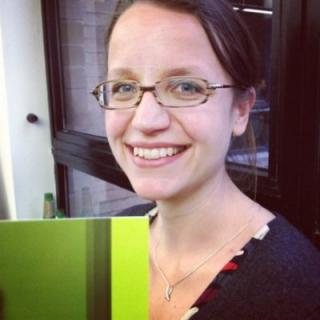
by Michael Manapat
Head of ML @Stripe
Stripe processes billions of dollars a year for businesses around the world. To protect its users from fraud, Stripe employs machine learning to detect potentially fraudulent transactions. In this talk, I'll describe how we bootstrapped this system and some of the most important aspects of industrial machine learning. We'll talk about how to choose, train, and evaluate models, how to bridge the gap between training and production systems, and how to address common pitfalls using the problem...
by Mathieu Bastian
Builds data products. @LinkedIn data science alumnus and @Gephi co-founder
Applied machine learning data pipelines are being developed at a very fast pace and often exceed traditional web/business applications codebase in terms of scale and complexity. The algorithms and processes these data workflows implement fulfill business-critical applications which require robust and scalable architectures. But how to make these data pipelines robust? When the number of developers and data jobs grow while at the same time the underlying data change how do we test that...
by Richard Kasperowski
Author of The Core Protocols: A Guide to Greatness
Open Space
by Michelle Lee
Data Ambassador, DataKind UK
People who repeatedly cycle through the criminal justice system, often for low-level crimes, cost the country millions of dollars in time and effort, but little is known about them. Who are these repeat offenders, and what drives their recidivism? Can we predict who they are to intervene before they enter the vicious cycle of recidivism?
The steps to build a predictive model may vary by project, but the fundamental workflow and principles remain the same. This talk will walk through...
by Cathy O'Neil
Author of the blog mathbabe.org
Algorithms are increasingly being used to automate what used to be human processes. They are potentially more fair and objective, but they are not automatically so. In fact they can easily codify unfair historical practices. I will discuss some examples of this problem and then I'll pose the question, how would we transparently and comprehensibly audit such algorithms for fairness?
by Emma Deraze
Lead data scientist @eRevalue
Natural Language Processing (NLP) is an essential part of a growing number of products, covering a vast scope of issues from the seemingly mundane (entity recognition) to the seriously involved (automatic summarization). In all cases, the goal is to reproduce or approximate a process - language - that we as humans rarely think twice about: this makes NLP a field fraught with misunderstandings and incorrect assumptions.
We will talk about the common pitfalls of treating language like '...
Tracks
Covering innovative topics
Monday, 7 March
-
Back to Java
What to expect in Java 9 and Spring 5
-
Stream Processing @ Scale
Big data, fast-moving data. Practical implementation lessons on Real-time Data
-
DevOps & CI/CD
Lessons/stories on optimizing the deployment pipeline
-
Head-to-Tail Functional Languages
Free-range Monads, Tackling immutability, tales from production, and more...
-
Architecting for Failure
Your system will fail. Take control before it takes you with it
-
21st Century Culture from Geeks on the Ground
New ways to organise technology companies and workplace culture
Tuesday, 8 March
-
Architectures You've Always Wondered about
In-depth technical case studies from giants like: Microsoft, Netflix, Google, Twitter, and more...
-
Close to the Metal
Get efficiency back into your code, concepts like: cache efficient algorithm and lock free data structures
-
Containers (in production)
Real-world lessons on scalability and reliability in production container deployments
-
Modern CS in the real world
Real-world Industry adoption of modern CS ideas
-
Security, Incident Response & Fraud Detection
Master-level classes on building security into your system and responding to incidents when things go wrong.
-
Optimizing You
Keeping life in balance is always a challenge. Learning lifehacks
Wednesday, 9 March
-
Disrupting Finance
Technology advances in finance (blockchain, P2P, Machine Learning, API's)
-
Modern Native Languages
Modern native languages: Safe efficiency with Go, Rust, Swift
-
Full Stack Javascript
Level up Javascript with topics like Angular, React/ReactNative, Node, Mongo/Couch/Other, Falcor, GraphQL, etc
-
Data Science & Machine Learning Methods
A developer's data science and machine learning toolkit
-
Microservices for Mega-Architectures
Practical lessons on Microservices success.
-
Modern Agile Development
Revisiting Agile today and tackling challenges we are seeing in the wild