Track Overview
AI/Machine Learning without a PhD
AI/ML is more approachable than ever. Discover how deep learning and ML is being used in practice. Topics include: TensorFlow, TPUs, Keras, PyTorch, & more. No PhD required.
From this track
How to Prevent Catastrophic Failure in Production ML Systems
AI systems can fail catastrophically and without warning, a characteristic not welcomed in the corporate environment. Martin will describe the unpredictable nature of artificial intelligence systems and how mastering a handful of engineering principles can mitigate the risk of failure. You’ll...
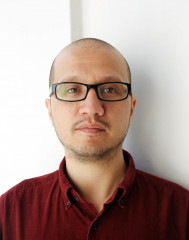
Martin Goodson
Chief Scientist/CEO @EvolutionAI
Test Driven Machine Learning
Software engineers are familiar with test driven development, but are not familiar with the statistical testing required in machine learning. Machine learning specialists are familiar with testing during the model building phase when they withhold data for cross-validation or final testing, but...
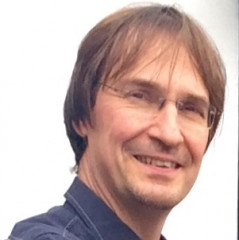
Detlef Nauck
Chief Research Scientist for Data Science @BTGroup and Visiting Professor @bournemouthuni
H2O's Driverless AI: An AI that creates AI
Through my kaggle journey to the top spot, I have noticed that many of the things I do as a data scientist can be automated. In fact automation is critical to achieve good scores and promote accountability, ensuring that common pitfalls in the modelling process are prevented. Through...
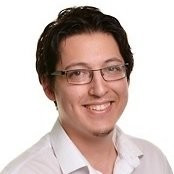
Marios Michailidis
Competitive Data Scientist @h2oai
Intuition & Use-Cases of Embeddings in NLP & Beyond
Machine Learning has achieved tremendous advancements in language tasks over the last few years (think of technologies like Google Duplex, Google Translate, Amazon Alexa). One of the fundamental concepts underpinning this progress is the concept of word embeddings (using something like the...
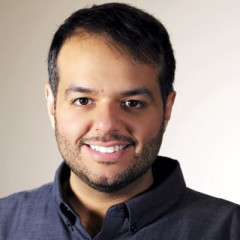
Jay Alammar
VC and Machine Learning Explainer @STVcapital
Understanding Deep Learning
No matter what your role is, it is really important to have some understanding of the models you’re working with. In last year's keynote, Rob Harrop talked about the importance of intuition in machine learning. This is a step towards that. You might already be using neural networks. How can...
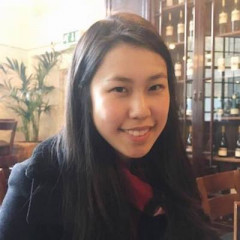
Jessica Yung
Machine Learning blogger and entrepreneur, Self-Driving Car Engineer Scholar @nvidia
AI/Machine Learning Open Space
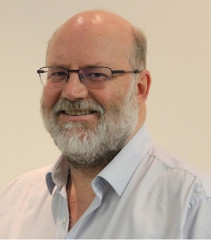
Shane Hastie
Director of Agile Learning Programs @ICAgile
Speakers from this track
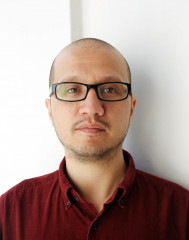
Martin Goodson
Chief Scientist/CEO @EvolutionAI
Martin Goodson is the chief scientist and CEO of Evolution AI, which develops a platform for large-scale natural language processing. Martin has designed machine learning products that are in use at FTSE 100 and Fortune 500 companies like Dun & Bradstreet, Time Inc., Royal Bank of Scotland,...
Read moreFind Martin Goodson at:
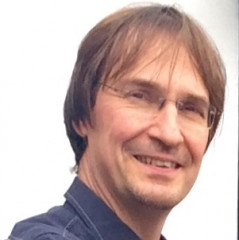
Detlef Nauck
Chief Research Scientist for Data Science @BTGroup and Visiting Professor @bournemouthuni
Detlef Nauck is Chief Research Scientist for Data Science with BT's Research and Innovation Division located at Adastral Park, Ipswich, UK. He is leading a group of international scientists working on research into Data Science, Machine Learning and AI. Detlef focuses on establishing best...
Read moreFind Detlef Nauck at:
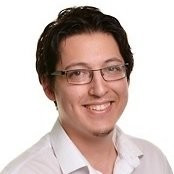
Marios Michailidis
Competitive Data Scientist @h2oai
Marios Michailidis is a competitive data scientist at H2O.ai currently working on Driverless AI – a software that automates machine learning. He holds a Bsc in accounting Finance from the University of Macedonia in Greece, an Msc in Risk Management from the University of Southampton and a...
Read moreFind Marios Michailidis at:
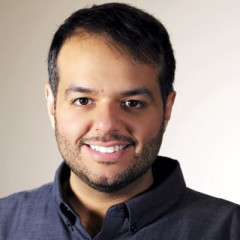
Jay Alammar
VC and Machine Learning Explainer @STVcapital
Through his blog and lessons on Udacity, Jay has helped tens of thousands of people wrap their heads around complex machine learning topics. Jay harnesses a visual, highly-intuitive presentation style to communicate concepts ranging from the most basic intros to data analysis, interactive intros...
Read moreFind Jay Alammar at:
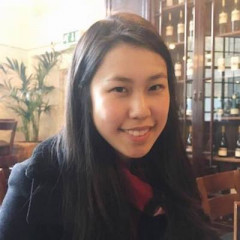
Jessica Yung
Machine Learning blogger and entrepreneur, Self-Driving Car Engineer Scholar @nvidia
Jessica is a research masters student in machine learning at University College London supervised by Prof. John Shawe-Taylor and André Barreto (Google DeepMind). She was previously at the University of Cambridge and was an NVIDIA Self-Driving Car Engineer Scholar. She applied machine learning to...
Read moreFind Jessica Yung at:
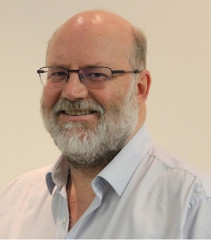
Shane Hastie
Director of Agile Learning Programs @ICAgile
Shane leads the Culture and Methods editorial team for InfoQ.com where he hosts the weekly InfoQ Culture Podcast. He is the Director of Agile Learning Programs for ICAgile. He has oversight in the strategic direction and expansion of ICAgile’s learning programs, including maintaining and...
Read moreFind Shane Hastie at:
Track Host
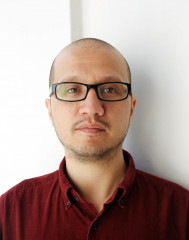
Martin Goodson
Chief Scientist/CEO @EvolutionAI
Martin Goodson is the chief scientist and CEO of Evolution AI, which develops a platform for large-scale natural language processing. Martin has designed machine learning products that are in use at FTSE 100 and Fortune 500 companies like Dun & Bradstreet, Time Inc., Royal Bank of Scotland,...
Read more