Track Overview
Machine Learning: The Latest Innovations
AI and machine learning is more approachable than ever. Discover how ML, deep learning, and other modern approaches are being used in practice.
From this track
BERT for Sentiment Analysis on Sustainability Reporting
Sentiment analysis is a commonly used technique to assess customer opinion around a product or brand. The data used for these purposes often consists of product reviews, which have (relatively) clear language and are even labeled (e.g. ratings). But when you look at what companies write about...
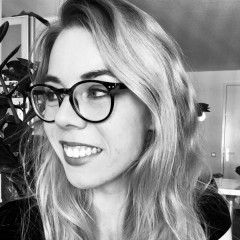
Susanne Groothuis
Sr. Data Scientist in the Advanced Analytics and Big Data team @KPMG
Accuracy as a Failure
When you see a green light, will you cross the street? Or will you still check for cars?When your machine learning model has demonstrated high accuracy, do you push it to production?This talk contains cautionary tales of mistakes that might happen when you let your data scientists on a goose...
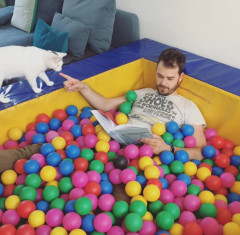
Vincent Warmerdam
Research Advocate @Rasa
Visual Intro to Machine Learning and Deep Learning
Break into machine learning with this gentle and intuitive journey through central concepts in machine learning -- from the most basic models up to the latest cutting edge deep learning models. This highly visual presentation will give you the mental map of ML prediction models and how...
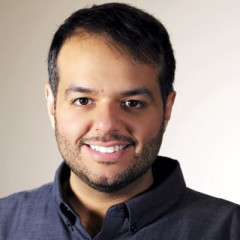
Jay Alammar
VC and Machine Learning Explainer @STVcapital
Speeding Up ML Development with MLFlow
Machine Learning is more approachable than ever before and the number of companies applying Machine Learning to build AI powered applications and products has dramatically increased in recent years. On this journey of adopting Machine Learning, many companies learn successful Machine...
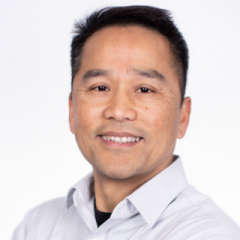
Hien Luu
Engineering Manager @LinkedIn focused on Big Data
Machine Learning Open Space
Details to follow.
The Fast Track to AI with Javascript and Serverless
Most people associate AI and Machine Learning with the Python language. This talk will explore how to get started building AI enabled platforms and services using full stack Javascript and Serverless technologies. With practical examples drawn from real world projects the talk will get you up and...
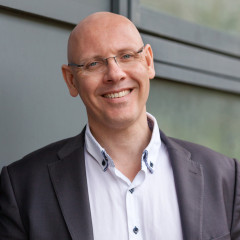
Peter Elger
Co-Founder & CEO @fourtheorem
Speakers from this track
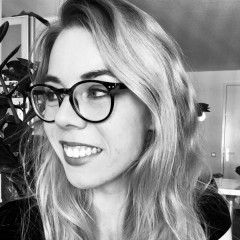
Susanne Groothuis
Sr. Data Scientist in the Advanced Analytics and Big Data team @KPMG
Susanne is a Data Scientist working in the Advanced Analytics and Big Data team of KPMG for the past three years. During her time at KPMG she has worked for clients in all different sectors such as governmental institutions, healthcare, transportation and others. In the most recent year she has...
Read moreFind Susanne Groothuis at:
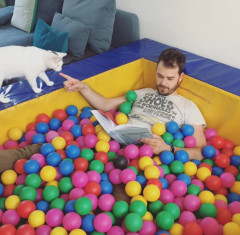
Vincent Warmerdam
Research Advocate @Rasa
My name is Vincent, ask me anything. I have been evangelizing data and open source for the last 6 years. You might know my from tech talks where I attempt to defend common sense over hype in data science. I currently work at Rasa.
Read moreFind Vincent Warmerdam at:
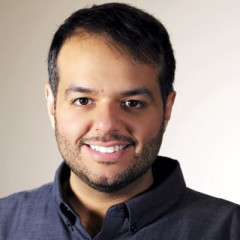
Jay Alammar
VC and Machine Learning Explainer @STVcapital
Through his blog and lessons on Udacity, Jay has helped tens of thousands of people wrap their heads around complex machine learning topics. Jay harnesses a visual, highly-intuitive presentation style to communicate concepts ranging from the most basic intros to data analysis, interactive intros...
Read moreFind Jay Alammar at:
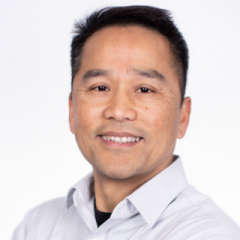
Hien Luu
Engineering Manager @LinkedIn focused on Big Data
Hien Luu is a technical lead of the Data Services Platform team at LinkedIn where he focuses on building big data infrastructure and big data applications. He loves working with big data technologies and recently became a contributor of Apache Pig project. He enjoys teaching and is currently an...
Read moreFind Hien Luu at:
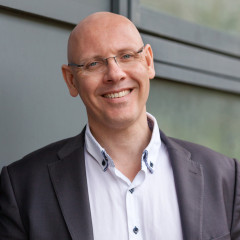
Peter Elger
Co-Founder & CEO @fourtheorem
Peter Elger is the co-founder and CEO of fourTheorem, a company providing expertise on next generation cloud architecture, agile development, AI and machine learning. Formerly a physicist working at the JET fusion research project, Peter was previously co-founder and...
Read moreTrack Host
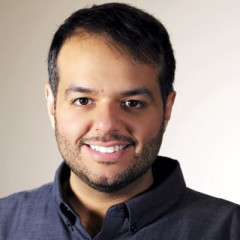
Jay Alammar
VC and Machine Learning Explainer @STVcapital
Through his blog and lessons on Udacity, Jay has helped tens of thousands of people wrap their heads around complex machine learning topics. Jay harnesses a visual, highly-intuitive presentation style to communicate concepts ranging from the most basic intros to data analysis, interactive intros...
Read more