MLOps has become a major enabler to successfully operationalize ML applications and for ML practitioners to realize the power of ML to bring impact to business. The journey to implementing MLOps will be unique to each company. One common thread to successfully adopt MLOps is the need for a strategy and a set of principles.
At DoorDash, we’ve been applying MLOps for a couple of years to support a diverse set of ML use cases, such ETA predictions, the Dasher assignments, personalized recommendation of restaurants and menu items and more, and to perform large scale predictions at low latency.
This session will share our approach to MLOps, the strategy and principles that have helped us to scale and evolve our platform to support hundreds of models and billions of predictions per day, and deep dive on the technical aspects of scaling our feature store and prediction service.
Interview:
What's the focus of your work these days?
I am currently leading the ML platform team at DoorDash. Our platform journey started a few years back and we are currently working on the second floor of our ML platform to support the various large and complex ML use cases that require distributed model training, model prediction flexibility and more
The use cases I mentioned are about recommendations, NLP, computer vision, and large language models.
What's something interesting that you've learned from the previous QCon?
At the previous QCon SF in 2022, one interesting thing I learned while attending the lightning talk given by Courtney Kissler, CTO @ Zuliy at the Women & Allies in Tech Breakfast was about using the criteria when making impactful decisions.
Speaker
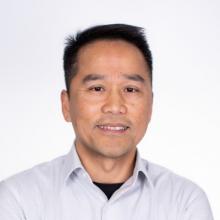
Hien Luu
Sr. Engineering Manager @Zoox & Author of MLOps with Ray, Speaker and Conference Committee Chair
Hien Luu is a Sr. Engineering Manager at Zoox, leading the Machine Learning Platform team. He is particularly passionate about building scalable AI/ML infrastructure to power real-world applications. He is the author of MLOps with Ray and the Beginning Apache Spark 3 book. He has given presentations at various conferences such as MLOps World, QCon (SF,NY, London), GHC 2022, Data+AI Summit, XAI 21 Summit, YOW Data!, appy()