Summary
Disclaimer: This summary has been generated by AI. It is experimental, and feedback is welcomed. Please reach out to info@qconlondon.com with any comments or concerns.
AI for Food Image Generation in Production: How & Why
This presentation explores the development lifecycle and implementation of a food image generation solution at Delivery Hero, highlighting the technical and business aspects of using AI for generating food images. Presented by Iaroslav Amerkhanov, it outlines the process from hypothesis to scalable deployment.
- Business Rationale: The project aims to improve menu content quality, hypothesizing that better content enhances conversion rates. Initial data showed that 86% of products with images had higher conversion rates, emphasizing the need for image generation over text descriptions.
- Technical Approach: The development involved creating a Minimum Viable Product (MVP) using Google Cloud Platform (GCP) and optimizing the image generation models for efficiency. Key components included text-to-image generation and in-painting techniques.
- Image Quality Control: Comprehensive quality checks ensured the consistency and appropriateness of generated images. This involved object detection, bounding box checks, and color adjustments using computer vision models.
- Production Scaling: The transition to production involved migrating models to self-hosted solutions to optimize costs. The deployment utilized NVIDIA GPUs and optimized stable diffusion models for better performance.
- Results and Impact: The solution generated over a million images, covering over 100,000 products, and achieved a 6-8% increase in conversion rates through A/B testing.
- Challenges and Solutions: Issues with non-Latin language support and large prompt processing were solved by translations and chunking methods. Also, a safety system was implemented for content validation to ensure image quality.
- Learnings: Key takeaways include the importance of infrastructure optimization and the need for robust quality measurement mechanisms before model fine-tuning. Avoiding cross-cloud implementations unless necessary was also advised.
Overall, this session illustrated the significant business impact and technical challenges of implementing AI-driven solutions in the food delivery sector, with a focus on generating high-quality visual content at scale.
This is the end of the AI-generated content.
Abstract
In this talk, we will conduct a technical overview of a client-facing Food Image Generation solution developed at Delivery Hero. We will explore step-by-step the stages of the product development cycle starting from an initial business hypothesis, following up with the fast-to-market product validation MVP and the full-scale productization phase that enabled generating 100,000 images per day.
The first focus of our discussion will be on the modern approaches in Image Generation that enable the generation of high-quality food-related visual content. We will explore the challenges of experimenting with the image generation models, the evaluation techniques and the ways to fine-tune these models. We'll also cover a set of advanced Computer Vision methods that help maintain high standards of visual content quality by automatic validation of the images across dimensions like positioning, colour balance, appropriateness and content relevance.
We will also consider the practical aspects of serving and scaling the visual models in production depending on the maturity level of the product and the infrastructure. Following up with the technical stack, we will outline the most appropriate approaches for each of the stages focusing on cost-efficiency, as well as the architectural decisions made at Delivery Hero to host and scale a zoo of visual models.
Interview:
What is the focus of your work?
As a Senior Data Scientist at Delivery Hero, I'm leading the AI-related projects of the AI Menu Content team. Our main products cover image generation and image enhancement problems. The development process includes several steps: data curation and labeling, modeling, experimentation, and integration into production. Our model zoo includes a set of Computer Vision and Image Generation methods based on the latest advancements in the area, which makes it highly fascinating to work with.
What’s the motivation for your talk?
The primary goal behind my speech is to motivate more teams in the industry to work on the image generation problem. That's why I'm excited to demonstrate the business case and the value such work could bring to a company.
Who is your talk for?
The talk targets AI & ML leaders and practitioners: we'll go through the business foundations and impact, following up with the methods used and the details on how the models are served in production. The focus will be on the applied part of the story and the real-world use cases.
What do you want someone to walk away with from your presentation?
With such rapid development in the area of deep learning and AI in general, many companies still wait for a broader adoption of these technologies in the industry before investing in them. The most important point I'd like to make clear is that with the modern stack of available frameworks, cloud services, and open-source models it's possible to achieve low time-to-market, making such investments justified.
What do you think is the next big disruption in software?
The AI agents which are capable of effectively utilizing the desktop setup and interacting with web services have the potential to disrupt the industry once their generalization capabilities and cost-efficiency reach a certain level. We can already see the first steps in this direction with the models from Anthropic and Open AI. It's obvious that the communication interfaces will evolve with time, potentially creating a new industry of AI-tailored operating systems and services.
Speaker
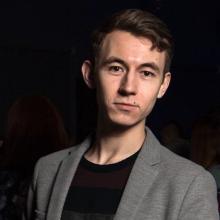
Iaroslav Amerkhanov
Senior Data Scientist @Delivery Hero, Founder of T4lky, Creator & Host of EPAM Podcast, Speaker
Iaroslav pioneered projects in Food Science at Delivery Hero and is now focused on generative AI solutions. He previously founded an EdTech startup and co-founded a sentiment analysis platform.