Gain a solid understanding of the fundamental concepts, tools, and techniques of artificial intelligence and machine learning, empowering software engineers to build intelligent and innovative applications.
From this track
Deploy MultiModal RAG Systems with vLLM
Tuesday Apr 8 / 10:35AM BST
While text-based RAG systems have been everywhere in the last year and a half, there is so much more than text data. Images, audio, and documents often need to be processed together to provide meaningful insights, yet most RAG implementations focus solely on text.
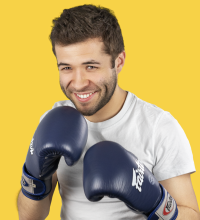
Stephen Batifol
Developer Advocate @Zilliz, Founding Member of the MLOps Community Berlin, Previously Machine Learning Engineer @Wolt, and Data Scientist @Brevo
How to Unlock Insights and Enable Discovery Within Petabytes of Autonomous Driving Data
Tuesday Apr 8 / 11:45AM BST
For autonomous vehicle companies, finding valuable insights within millions of hours of video data is essential yet challenging.
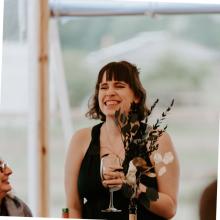
Kyra Mozley
ML Engineer @Wayve, Previously Security & AI PhD Candidate @Royal Holloway University
AI for Food Image Generation in Production: How & Why
Tuesday Apr 8 / 01:35PM BST
In this talk, we will conduct a technical overview of a client-facing Food Image Generation solution developed at Delivery Hero.
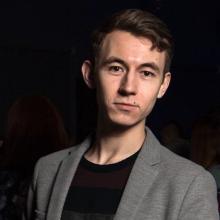
Iaroslav Amerkhanov
Senior Data Scientist @Delivery Hero, Founder of T4lky, Creator & Host of EPAM Podcast, Speaker
Foundation Models for Recommenders: Challenges, Successes, and Lessons Learned
Tuesday Apr 8 / 02:45PM BST
Recommender systems are an integral part of most products nowadays and are often a key driver of discovery for users of the product.
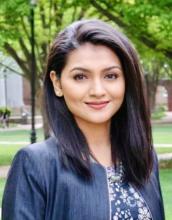
Moumita Bhattacharya
Senior Research Scientist @Netflix, Previously @Etsy
Building Embedding Models for Large-Scale Real-World Applications
Tuesday Apr 8 / 03:55PM BST
Embedding models are at the core of search, recommendation, and retrieval-augmented generation (RAG) systems, transforming data into meaningful representations.
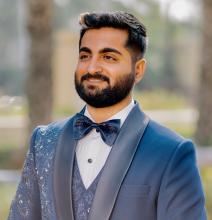
Sahil Dua
Senior Software Engineer, Machine Learning @Google, Stanford AI, Co-Author of “The Kubernetes Workshop”, Open-Source Enthusiast
Lessons Learned From Building LinkedIn’s First Agent: Hiring Assistant
Tuesday Apr 8 / 05:05PM BST
In October 2024, we announced LinkedIn’s first agent, Hiring Assistant to a select group of LinkedIn customers.
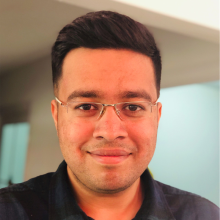
Karthik Ramgopal
Distinguished Engineer & Tech Lead of the Product Engineering Team @LinkedIn, 15+ Years of Experience in Full-Stack Software Development
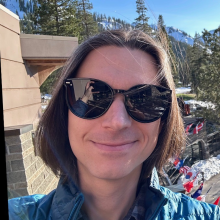
Daniel Hewlett
Principal AI Engineer & Technical Lead for AI @LinkedIn, 12+ Years of Expierence in ML and AI Engineering, Previously @Google